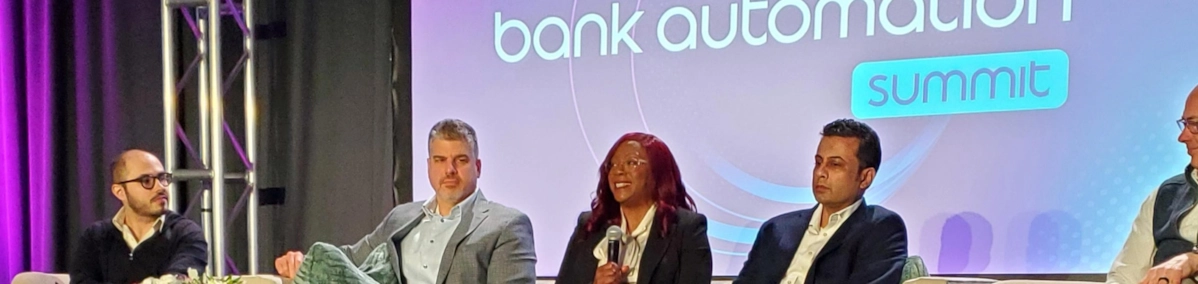
I had the pleasure of speaking on a panel at the Bank Automation Summit in Nashville. One of my panel questions asked about the common friction points among clients when implementing AI. Like it or not, Artificial Intelligence (AI) is transforming industries by optimizing processes, improving decision-making, and unlocking new possibilities. However, the path from AI adoption to full-scale success is rarely straightforward. Many organizations encounter challenges that hinder progress, create misunderstandings, and lead to ineffective implementations. Here are the common challenges I discussed—along with strategies to overcome them.
1. The Expectation-Execution Gap
One of the biggest hurdles in AI implementation is the disconnect between leadership expectations, execution realities, and the ongoing maintenance required. Many executives believe AI is a plug-and-play solution that will instantly resolve business challenges, while employees fear AI will replace them. In reality, AI requires careful planning, iterative refinement, and human oversight to function effectively.
How to Address It:
- Educate stakeholders about AI's capabilities and limitations to set realistic expectations.
- Position AI as an augmentation tool rather than a replacement, emphasizing how it enhances productivity rather than eliminates jobs.
- Create a structured change management plan to support employees as AI is introduced, ensuring they understand their evolving roles.
- Over-reliance —believing AI outputs are infallible and making decisions without human validation.
- Under-reliance —dismissing AI insights due to a lack of trust or understanding.
- Implement "human-in-the-loop" validation, ensuring experts review AI-generated decisions.
- Provide training for employees interacting with AI, so they understand how AI-derived insights fit into their decision-making processes.
- Develop clear guidelines for AI validation and usage, ensuring the organization balances automation and human judgment.
- Establish a structured AI discovery process, similar to traditional business process optimization.
- Focus on AI opportunities that generate revenue, reduce risk, or enhance customer experience.
- Involve cross-functional teams (business leaders, data scientists, IT, and end-users) in the AI discovery phase to ensure practical, high-impact use cases are selected.
- Conduct a data audit before AI implementation to assess data quality.
- Invest in data cleansing and governance initiatives to ensure AI models can access accurate and complete data.
- Use feedback loops to continuously improve AI models and update them as new, higher-quality data becomes available.
2. The Over- and Under-Reliance on AI-Assisted Decisions
AI can significantly improve decision-making, but companies often fall into one of two traps:
Both scenarios are problematic. AI models are only as good as the data they’re trained on, and without human oversight, errors can go unnoticed. On the other hand, failing to leverage AI insights reduces the value AI can bring.
How to Address It:
3. The Need for a Structured AI Discovery Process
AI adoption should be as strategic as automation efforts. Early automation initiatives often involved handpicking opportunities without a structured framework, leading to inefficiencies. The same mistake is being repeated with AI—organizations invest in AI without a systematic approach to identifying where it will drive real value.
How to Address It:
4. AI’s Dependence on High-Quality Data
AI models thrive on data—but the results will be unreliable if that data is incomplete, inconsistent, or biased. Many organizations struggle with data silos, outdated records, and poor data governance, which can undermine AI performance.
How to Address It:
Implementing AI is not just a technical challenge—it’s an organizational transformation that requires strategic planning, clear communication, and ongoing training. By addressing these friction points early, businesses can set themselves up for AI success, ensuring their AI initiatives drive real value rather than becoming expensive, under-utilized projects.
As AI continues to evolve, companies that embrace a structured approach, invest in data quality, and empower employees to work alongside AI will gain the most competitive advantage. The key is not just adopting AI—but integrating it to align with business needs and human expertise.
Share this
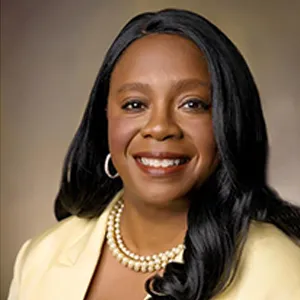
Dr. Danielle Jennings
Primus Practice Director, Intelligent Automation
Dr. Danielle Jennings serves as the Client Services Executive and Automation Practice Lead at Primus Software Corporation. In this dynamic role, she spearheads client engagement initiatives while contributing to the division’s growth and success. With 15 years of experience in IT, including 7 years specializing in intelligent automation, Dr. Jennings has been instrumental in implementing transformative technologies such as RPA, chatbots, AI, and ML in mid-size to Fortune 500 companies..