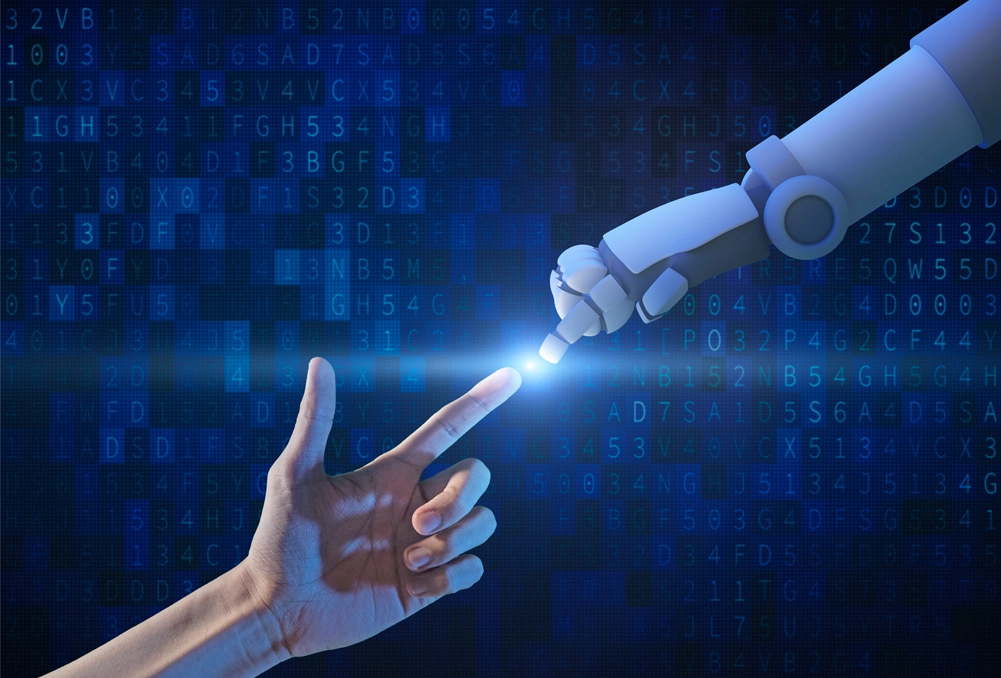
Agentic AI: A Paradigm Shift in Autonomous Intelligence
Abstract
Artificial Intelligence (AI) has evolved from simple rule-based systems to advanced generative models and, more recently, Agentic AI. Unlike Traditional AI, which follows predefined instructions, or Generative AI, which synthesizes content based on learned patterns, Agentic AI actively pursues complex goals with minimal human supervision. This white paper explores the key distinctions of Agentic AI, its benefits, and challenges with governance and calculating the return on investment (ROI).
Introduction
Artificial Intelligence (AI) has transformed industries by increasing efficiency and productivity, enhancing data analysis and decision-making, improving accuracy and reducing errors, and optimizing operations. However, AI systems are designed to allow humans to solve more complex problems. The emergence of Agentic AI marks a shift toward autonomous intelligence, where AI adapts, strategizes, and executes complex tasks with minimal oversight (Acharya et al., 2025). This paper contrasts Agentic AI, Traditional AI, and Generative AI, highlighting its potential benefits and challenges related to governance and ROI assessment.
Understanding Agentic AI
Agentic AI is characterized by autonomy, goal-directed behavior, and adaptability. Unlike Traditional AI, which operates within fixed guidelines, or Generative AI, which produces content based on trained data or adaptive insights, Agentic AI exhibits decision-making capabilities, environmental awareness, and goal-driven execution (Shavit et al., 2025).
Comparison with Traditional AI and Generative AI
Feature | Traditional AI | Generative AI | Agentic AI |
Function | Executes specific tasks | Generates new content | Achieves long-term goals autonomously |
Decision-Making | Rule-based, deterministic | Pattern-based synthesis | Context-aware, adaptive |
Autonomy | Low | Medium | High |
Use Cases | Predictive analytics, automation | Content creation, simulation | Robotics, dynamic systems, complex workflows |
Traditional AI relies on supervised learning and explicit programming (Mäntymäki et al., 2022). Generative AI creates new content by analyzing learned patterns and existing data. In contrast, Agentic AI integrates adaptability, goal-setting, and strategic execution, making it suitable for high-stakes, dynamic environments (Acharya et al., 2025).
Key Benefits of Agentic AI
Agentic AI presents several advantages over existing Artificial Intelligent frameworks, including efficiency, scalability, and adaptability.
- Autonomy & Task Complexity Handling
Agentic AI systems can self-optimize and navigate unpredictable conditions without human input. In finance, autonomous trading agents dynamically adjust strategies based on market fluctuations, outperforming rigid, rule-based trading bots (Shavit et al., 2025). - Enhanced Decision-Making & Context Awareness
Unlike Traditional AI, which operates within a predefined system (i.e., structured workflow), Agentic AI recognizes shifts in data, rules, or conditions and adapts its strategy to achieve the goal. In healthcare, AI-powered diagnostic systems can prioritize patient treatments based on real-time data analysis (Gianni et al., 2022). - Resource Optimization & Human-AI Collaboration
By autonomously allocating resources and adjusting strategies, Agentic AI reduces operational costs and allows human experts to focus on higher-value tasks. AI-powered predictive maintenance can preemptively repair machinery in manufacturing, preventing costly failures (Shavit et al., 2025).
Challenges in Agentic AI Deployment
Despite its advantages, Agentic AI introduces significant challenges, particularly in ROI assessment and ethical goal-setting.
Identifying ROI in Agentic AI
Unlike Traditional AI, which has clear efficiency metrics, Agentic AI’s value is often difficult to quantify due to:
- Unclear Performance Benchmarks: Defining success in dynamic, multi-objective environments is complex.
- Indirect Benefits: Increased efficiency and decision-making quality may not translate directly into financial ROI.
- Implementation Costs: High computational power and ethical compliance requirements add to deployment expenses.
A structured ROI framework is needed to evaluate financial, operational, and strategic benefits while accounting for long-term adaptability and risk mitigation (Mäntymäki et al., 2022).
Goal Setting Across Diverse Social Morals & Human Objectives
Agentic AI must align with human values, but defining universally acceptable goals is challenging due to:
- Unclear performance benchmarks: Diverse social and cultural norms: What is ethical in one region may not be in another.
- Conflicting Stakeholder Interests: Organizations, governments, and individuals may prioritize different objectives.
- Lack of Regulatory Clarity: Governance frameworks for autonomous AI decision-making remain underdeveloped (Gianni et al., 2022).
Ethical AI governance must incorporate public input, transparency, and adaptability to balance autonomy with human oversight (Shavit et al., 2025).
Ethical & Governance Considerations
Effective governance frameworks are necessary to ensure that Agentic AI aligns with societal values.
Responsible AI Development & Oversight
Governance frameworks should enforce:
- Transparency and Explainability: AI decisions must be interpretable and auditable.
- Ethical Guardrails: AI should align with legal, moral, and safety standards.
- Human-in-the-loop Oversight: Ensuring AI acts within acceptable boundaries (Gianni et al., 2022).
Global Standards for Ethical AI Deployment
As AI adoption grows, global regulatory bodies must standardize policies to:
- Prevent Misuse: Address concerns of bias, security, and accountability.
- Enable Interoperability: Ensure AI compliance across international markets.
- Encourage Innovation: Balance risk management with technological progress (Mäntymäki et al., 2022).
Conclusion & Future Outlook
Agentic AI represents a transformative shift in AI capabilities, enabling autonomous, goal-driven intelligence. However, its successful adoption requires:
- Clear ROI measurement frameworks.
- Ethical goal-setting mechanisms that align with human objectives.
- Robust governance models to ensure safe and responsible deployment.
Future research should focus on developing AI accountability structures, enhancing AI-human collaboration, and refining adaptive learning models. If properly governed, Agentic AI has the potential to revolutionize industries while preserving ethical integrity.
References
- Acharya, D. B., Kuppan, K., & Divya, B. (2025). Agentic AI: Autonomous Intelligence for Complex Goals—A Comprehensive Survey. IEEE Access. DOI:10.1109/ACCESS.2025.3532853.
- Gianni, R., Lehtinen, S., & Nieminen, M. (2022). Governance of Responsible AI: From Ethical Guidelines to Cooperative Policies. Frontiers in Computer Science. DOI:10.3389/fcomp.2022.873437.
- Mäntymäki, M., Minkkinen, M., Birkstedt, T., & Viljanen, M. (2022). Defining Organizational AI Governance. AI and Ethics, 2(603-609).
- Shavit, Y. et al. (2025). Practices for Governing Agentic AI Systems.
Share this
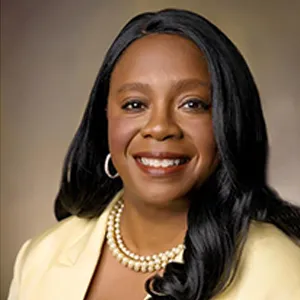
Dr. Danielle Jennings
Primus Practice Director, Intelligent Automation
Dr. Danielle Jennings serves as the Client Services Executive and Automation Practice Lead at Primus Software Corporation. In this dynamic role, she spearheads client engagement initiatives while contributing to the division’s growth and success. With 15 years of experience in IT, including 7 years specializing in intelligent automation, Dr. Jennings has been instrumental in implementing transformative technologies such as RPA, chatbots, AI, and ML in mid-size to Fortune 500 companies..